Data Quality + Data Governance Frameworks
Data Governance is the foundation of your data strategy. It will give your business the maximum value from your data, by ensuring data quality; the data that you use to make data-driven business decisions, will always be reliable, consistent and trustworthy.
In this article we’ve covered the most important areas of data governance: what it is and why it is important:
> Tailored Data Quality Framework/ Data Governance Framework
> Roles and Responsibilities for Working Groups and Data Governance Councils
> Consent management
> Ethics frameworks
> Data Governance Assessments
> Metadata management
> Advanced Data Governance capabilities
> Data quality assessments and remediation
> Data security and compliance
> Bespoke Data Governance Framework
Tailored Data Governance Framework: What is it?
A customised workflow and methodology used to monitor, manage, remediate data within an organisation. This workflow and methodology within an organisation look similar to the below.
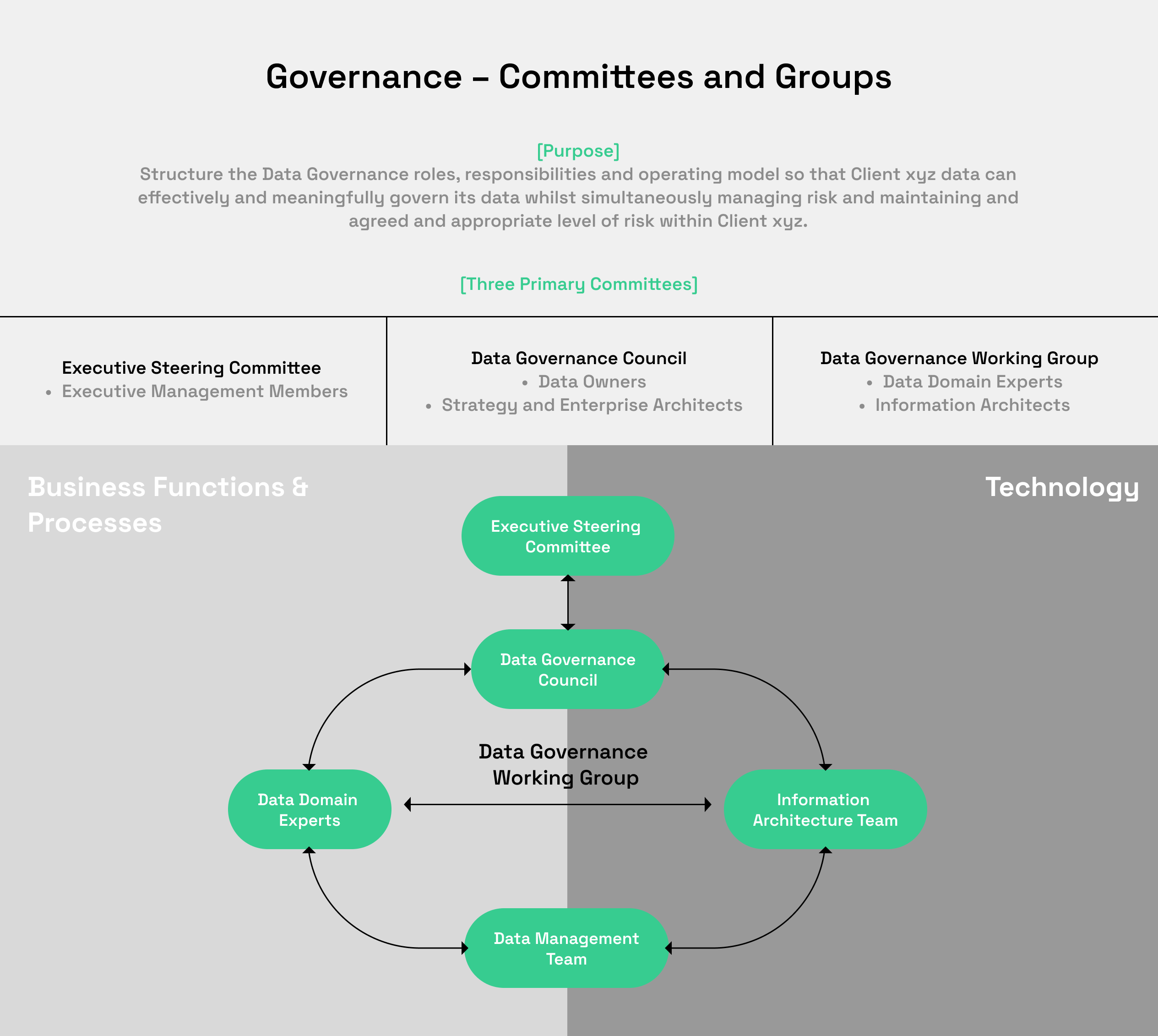
An established way of working incorporates a customised set of rules and guidelines to manage and protect your data effectively.
The responsibilities of those roles within the data quality framework specifies how the data should be categorised, who can access them, how they should be stored, how long they should be stored for and what should happen if something goes wrong.
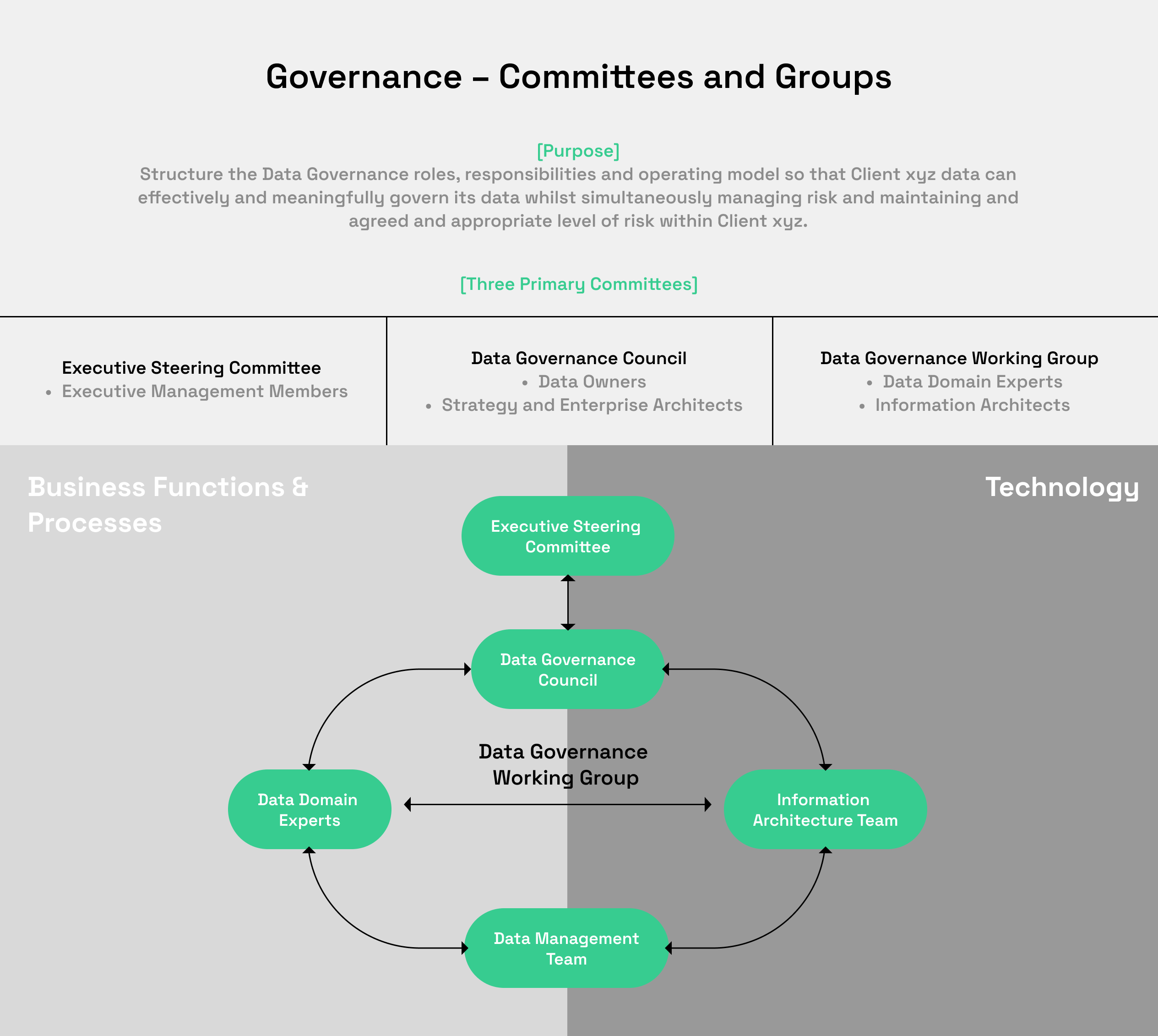
The responsibilities of those roles within your framework will also specify:
- Which sensitive data should be encrypted and only accessible to authorised individuals.
- Who is responsible for managing the data and ensuring their accuracy.
- Outline processes for data backup, data retention, and data disposal.
Data Quality Framework: Why have it?
- Having a data governance framework in place, gives you peace of mind.
- You want to make sure that all the data that your organisation produces is well organised, secure, and easily accessible to the right people.
- It ensures that your data is managed in a consistent and secure manner. It helps you to avoid data breaches, comply with regulations.
- You know that the resulting data quality will allow you to make informed decisions based on reliable information.
- The same terms/metrics are derived from the same dataset, defined and calculated across the business consistently.
- Accountability and responsibility for looking after and managing your data is clear
Roles and Responsibilities for Working Groups and Data Governance Councils
Notitia can assist your team with creating roles and responsibilities for working groups and data governance councils, so that your business can effectively manage and make decisions about your data and ensure that it is handled properly.
These roles are clearly defined and communicated within your business so people know who does what and when as well as what to expect from who, when and why.
Overall, the roles and responsibilities within working groups and data governance councils ensure that different aspects of data management are addressed, decisions are made collectively, and data is handled in a responsible and organised manner throughout your business.
What is a working group?
Working groups form smaller teams that focus on specific aspects of data governance. They might be responsible for tasks such as data quality, data privacy, or data security. Each person in the working group has a specific role, like being in charge of data analysis, data documentation, or data access control.
The Working group instigates defining, creating, monitoring and managing exception reports to help with monitoring data quality.
What is a data governance council?
A data governance council is a group that oversees the overall data governance strategy and makes important decisions about data management. They might set policies and guidelines, review data-related issues, make decisions on how to handle them and resolve issues that cannot be resolved by the Working Group and are escalated to the Council. The council usually consists of senior leaders or representatives from different departments/business units within your organisation.
The roles and responsibilities within these groups can vary, but some common examples include:
>Data Stewards: Responsible for ensuring the quality, accuracy, and integrity of data. They may define data standards, resolve data-related issues, and enforce data policies.
>Data Analysts: Analyse data and provide insights to support decision-making. They may create reports, perform data analysis, and identify trends or patterns in the data.
>Data Privacy Officers: Focus on protecting personal and sensitive data. They ensure compliance with privacy laws and regulations, manage data access permissions, and handle data breach incidents.
>IT Administrators: Manage the technical aspects of data governance, such as data storage, data backups, and data security. They may implement data management tools and technologies and provide technical support to the data governance initiatives.
Consent management
Consent management is the process of obtaining and managing the permissions or approvals from individuals regarding the use of their personal information or participation in certain activities.
Businesses collect and use personal data for various purposes, such as sending marketing emails, analysing user behaviour, or sharing data with third parties.
Not only is it important to respect individuals' privacy and give them control over their data, but in Australia, there is legislation in place to protect the rights of consumers such as The Privacy Act and SPAM Regulations 2021.
By implementing effective consent management, businesses and organisations can build trust with individuals, ensure compliance with privacy regulations, and demonstrate a commitment to protecting personal data. It empowers individuals to have control over their information and promotes responsible data handling practices.
Ethics framework
An ethics framework is compiled of guidelines and principles that ensure ethical and responsible use of data.
These rules will help your business to make fair and moral decisions when it comes to handling and use of data. The framework is designed to protect people's rights, prevent harm, and promote transparency and trust.
Businesses and organisations collect, store, and analyse vast amounts of data, which is only set to increase.
It’s important to use this data in a way that respects people's privacy, avoids discrimination, and maintains fairness. Ethics frameworks provide a framework to do just that.
By following an ethics framework in data governance, your business will foster trust with individuals, uphold ethical standards, and navigate the complex landscape of data in a responsible and conscientious manner.
It helps ensure that data is used to benefit society, while respecting the rights and well-being of individuals.
What are the components of an ethics framework in data governance?
An Ethics Framework involves privacy and consent, fairness and non-discrimination, transparency and accountability, data security and integrity, social and environmental impact.
Data Governance Assessments
Data governance assessments are evaluations or audits conducted to measure and understand how well a business manages and governs its data. During a data governance assessment, experts or professionals review various aspects of data management within an organisation. They examine processes, policies, and practices related to data to identify strengths, weaknesses, and areas for improvement.
Data governance assessment outcomes
Based on our findings, we will provide your business with tailored recommendations or action plans that address any identified weaknesses or gaps in data governance. These recommendations may include process improvements, policy enhancements, training initiatives, or technology implementations.
An independent data governance assessment will provide you with an outside perspective, based on the most up-to-date recommendations. It will consider your current state of data management, identify areas for improvement, and develop strategies to enhance data governance practices.
What are the steps in a data governance assessment?
1. Data Policies and Procedures: Do you have well-defined policies and procedures in place for data management? This includes aspects such as data quality, data privacy, data security, and data retention, how often the working group meets, how often are Data Quality and Completeness Exception reports determined, run and reviewed?
2. Data Organisation and Classification: How effectively is your data organised, classified, and labelled? This involves assessing the effectiveness of data categorisation, naming conventions, and metadata management.
3. Data Access and Permissions: How is data access controlled in your business? Are there appropriate access controls in place?Is access to sensitive or confidential data properly restricted to authorised individuals?
4. Data Quality and Integrity: The accuracy, completeness, and reliability of your data. Have data quality controls been appropriately implemented? Have you implemented mechanisms to monitor and address data issues?
5. Data Governance Roles and Responsibilities: How are the data governance responsibilities assigned and understood by your people? Are there clear roles and accountabilities for data management and data governance? Do you have an appropriate leadership and governance structure in place including a proactive and visible business sponsor?
6. Compliance and Legal Requirements: Does your business comply with relevant data protection laws, regulations, and industry standards? What are your efforts to protect individual privacy rights and maintain legal and ethical data handling practices?
Metadata management
Metadata management is the practice of organising and managing information about data. It's like having a catalogue or index that helps you understand and find the data you have.
Businesses and organisations need to manage their vast amounts of data stored in various systems and databases.
Metadata provides additional information about that data, such as its meaning, structure, origin, and context. It helps users understand what the data represents and how it can be used effectively.
Effective metadata management improves data understanding, facilitates data sharing and collaboration, and enhances the overall data governance and data management processes.
It makes it easier for your people to locate, analyse, and use data, leading to more informed decision-making and better business outcomes.
Business + Tech Data Dictionary
A data dictionary is a structured document or repository that provides a comprehensive description of the data elements and attributes used in a database or information system
It includes information about the data's meaning, relationships, formats, constraints, and usage. And is a valuable reference for data analysts, database administrators, and developers to understand and manage the data effectively
You need a data dictionary that is easy for your people to locate. It’s also important to assign roles that have accountability for managing and updating, so that the content remains current and relevant.
Here are the key aspects of your data dictionary:
1. Data Description: Characteristics and properties of data. It includes information like data type, field names, data formats, units of measurement, and data relationships. This descriptive metadata helps users interpret and use the data correctly.
2. Data Lineage: Metadata can track the origin and history of data. It captures information about the source systems, data transformations, and any modifications made to the data over time. Data lineage helps users understand the reliability, credibility, and trustworthiness of the data.
3. Data Access and Permissions: It includes information about data access permissions and security. It specifies who can access the data, what level of access they have, and any restrictions or confidentiality requirements associated with the data.
4. Data Governance: Metadata management plays a crucial role in data governance initiatives. It helps organisations enforce data standards, policies, and compliance requirements. By maintaining metadata about data ownership, data usage, and data classification, businesses ensure that data is handled appropriately and in accordance with Australian regulations and legislation.
5. Data Discovery and Search: By indexing and cataloguing metadata, users can quickly find relevant data based on specific criteria or keywords. This simplifies the process of locating and accessing the right data for analysis, reporting, or decision-making purposes.
6. Data Integration and Interoperability: Metadata management facilitates data integration by providing information on how different data sets relate to each other. It helps identify common data elements, establish data mappings, and ensure data interoperability across systems and applications.
Advanced Data Governance “Lifecycle” capabilities
Advanced data governance capabilities refer to a set of practices and tools that ensure your data is properly managed, controlled, and maintained throughout its lifecycle.
It includes proactive data ownership, data stewardship, regular monitoring and issue resolution, adherence to good data quality, monitoring key indicators and retiring/decommissioning of data when it is no longer required which, in turn, mitigates the risks associated with data and cybersecurity.
By implementing advanced data governance capabilities, your business can proactively manage your data assets, ensure data quality and compliance, and mitigate risks associated with data-related issues. This leads to improved decision-making, increased operational efficiency, and enhanced trust in your data.
Proactive Data Ownership:
Proactive data ownership means assigning clear responsibility to individuals or teams within an organisation or business, for the quality, accuracy, and compliance of the data. It involves establishing accountability and ensuring that someone is actively monitoring and managing the data.
Data Stewardship:
Data stewardship involves the ongoing management and protection of data assets. Data stewards are responsible for defining and enforcing data quality standards, ensuring data is classified and labelled correctly, and resolving any data-related issues that arise. They act as guardians of the data and oversee its proper handling, as well as being key contact points for users of data across the business.
Regular Monitoring and Issue Resolution:
Regular monitoring involves keeping a close eye on the data to identify any issues or anomalies. This could include data inconsistencies, errors, or breaches. Once identified, appropriate steps are taken to investigate, resolve, and remediate these issues to maintain data integrity. Exception reports are a key component supporting this activity.
Adherence to Good Data Quality:
Good data quality refers to data that is accurate, complete, consistent, and reliable. Advanced data governance capabilities include defining standards and mechanisms to ensure adherence to these quality standards. This involves implementing data validation processes, data cleansing techniques, and data quality metrics to measure and improve the overall quality of the data.
Monitoring Key Indicators:
Success or failure of data governance can be evaluated by monitoring key indicators or metrics. These indicators could include data accuracy rates, data completeness, compliance with data regulations, response time for issue resolution, and user satisfaction with data quality. Monitoring these indicators helps a business to identify areas of improvement and measure the effectiveness of their data governance efforts. These data governance metrics need to be referred to in your whole-of-business strategy.
Data quality assessments and remediation
Data quality assessments and remediation is the process of evaluating the quality of your business data and taking necessary actions to improve or fix any issues found.
By conducting data quality assessments and remediation, your business can proactively identify and resolve data issues before you introduce a change. This ensures that your data remains accurate, consistent, and reliable.
Here’s an example of impact analysis on data quality when a change is introduced:
Company (A) uses a customer relationship management (CRM) system to store and manage customer information. They want to start collecting additional data from their customers.
Before implementing this change, Company (A) needs to assess the impact on data quality.
1. Impact Analysis: Company (A) conducts an impact analysis to understand how the new feature will affect existing data and data quality. They assess how the new data elements will fit into the existing data model and determine if any changes are required to accommodate the new data.
Here, you also need to consider Business Process amendments, communication of the changes and their business benefits to users of the application and users of the data captured by that application.
This would (ideally) be communicated by the business sponsor of that initiative to try and mitigate the risk of the change being seen as "another bl**dy IT thing!"
Consider how the new data will be captured, stored, and integrated with other systems, along with assigning a Data Owner and Data Steward to additional data.
2. Data Quality Assessment: In the context of this example, data quality assessment involves evaluating the accuracy, completeness, consistency, and relevancy of the customer data. Company (A) examines the existing customer data in the CRM system to identify any potential issues that may arise due to the system amendment. They may look for missing or duplicate records, inconsistencies in data formats, or any other data anomalies.
3. Data Remediation: Based on the data quality assessment, Company (A) takes remedial actions to address the identified data issues. For instance, they may cleanse the data by removing duplicates or filling in missing information. They may also implement data validation rules to ensure that the new data captured is accurate and consistent. Additionally, they may update data integration processes to accommodate the changes and maintain data integrity across different systems.
4. Ongoing Monitoring: Once the system amendment is implemented, Company (A) continues to monitor data quality to ensure that the changes have not introduced any new issues. They regularly assess data quality metrics, such as error rates or data completeness, to identify and resolve any emerging issues promptly. Ongoing monitoring helps maintain the overall quality of the data and ensures that the system amendment does not negatively impact data integrity.
Data security and compliance
Data security and compliance, under the umbrella of data governance, refer to practices and measures put in place to protect data from unauthorised access, ensure data privacy, and adhere to relevant regulations and standards. It involves Data Lifecycle Management, proactive data security measures and compliance with regulatory cyber security and privacy requirements.
Here’s an example of proactive security of data through Data Lifecycle Management (DLM):
Imagine that “healthcare organisation (A)” handles sensitive patient information.
They have implemented a Data Lifecycle Management policy to ensure the data security, privacy and regulatory compliance of patient data throughout its lifecycle. This not only safeguards sensitive data but also fosters trust among patients and stakeholders, knowing that their information is handled securely and in compliance with relevant standards.
1. Data Lifecycle Management: Data Lifecycle Management involves managing data from its creation or acquisition to its eventual deletion or archival. It includes various stages, such as data creation, storage, usage, sharing, and disposal. It establishes a framework for managing data securely and in accordance with industry regulations and best practices.
2. Proactive Security: Proactive security means taking preventive measures to protect data from potential threats. In the context of the example, healthcare organisation (A) implements measures such as access controls, encryption, and regular security audits to safeguard patient data. These measures are put in place before any security breach occurs, aiming to prevent unauthorised access or data breaches.
3. Data Security Measures: healthcare organisation (A) implements various security measures as part of DLM. They enforce strong user authentication to control access to patient data. They encrypt data both at rest (stored on servers or databases) and in transit (when data is being transmitted over networks). Regular security audits and vulnerability assessments may also be conducted to identify and address any potential weaknesses in the system.They also implement proactive monitoring of when their data is no longer required to be stored (one of many issues uncovered by recent Australian data breaches).
4. Compliance with Regulations: In the healthcare sector, there are specific regulations that govern the protection of patient data. By complying with these regulations, the healthcare organisation (A) ensures that they meet the legal requirements for data security and privacy. This includes implementing appropriate security controls, maintaining audit logs, and conducting regular risk assessments.
>Questions about how we can help? Book a meeting.
About Alex Avery, Notitia Managing Director + Founder
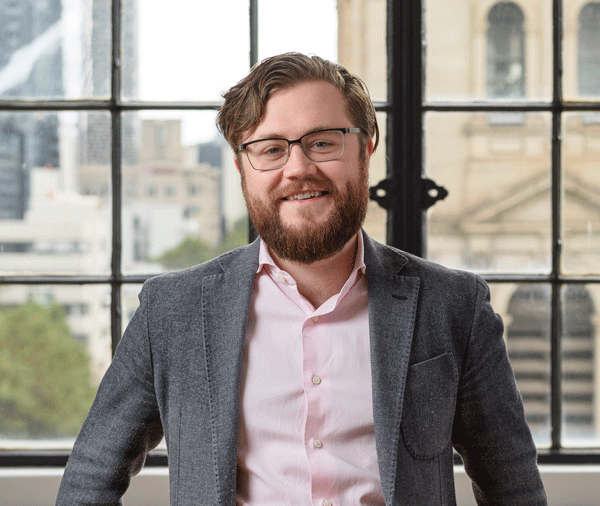
Alex Avery, Notitia Founder and Managing Director, heads our operations in Melbourne to provide data analytics and digital transformation services to more than 60 clients across Australia.
He is highly regarded for his ability to quickly analyse complex operational scenarios and provide workable solutions to achieve business objectives. This, along with considerable experience in the management of technical and business teams across both project environments and "business as usual" is the reason why most clients have come via word of mouth.
Having spent close to a decade working across Australian and global startups, Big 4 consulting and academia, Alex launched Notitia in 2019, which has since skyrocketed in growth.
Today, Alex leads his expanding team across data + analytics, design + development + strategy to help Australian clients solve their data challenges.
He sees technology as an enabler and partners with the biggest vendors to utilise best of breed software.
With the right technology and expertise, Alex says that problems of any size can be solved through valuable insights from accurate data.
Book a chat with Alex to find out how he can solve your data challenge.